

In Proceedings of the 41st International ACM SIGIR Conference on Research & Development in Information Retrieval (SIGIR’18). Relating personality types with user preferences in multiple entertainment domains.
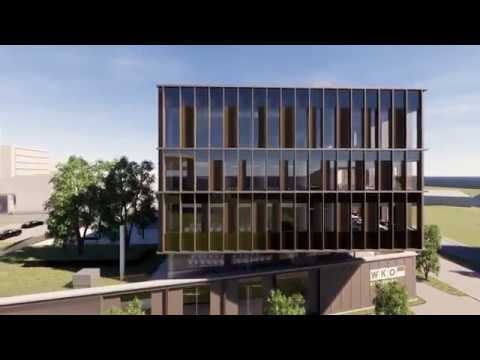
In Proceedings of the 1st International Workshop on Decision Making and Recommender Systems (DMRS’14). On the exploitation of user personality in recommender systems. Hybrid recommender systems: Survey and experiments. Springer New York, New York, NY, 1073–1080. Measuring emotion: The self-assessment manikin and the semantic differential. Team gender diversity and investment decision making behavior. Personality-based recommendation in E-commerce. De Vivo, Matteo Gaeta, Giuseppe Sansonetti, and V. In Proceedings of the 4th ACM Conference on Recommender Systems (RecSys’10). Group-based recipe recommendations: Analysis of data aggregation strategies.
#H graz servicecenter movie#
Emotion-based movie recommendations: How far can we take this? In Proceedings of the 3rd Workshop on Emotions and Personality in Personalized Systems (EMPIRE’15). Experimental evaluation of context-dependent collaborative filtering using item splitting. Enhancing group decision making: An exercise to reduce shared information bias. Association for Computing Machinery, New York, NY, 24–32. In Proceedings of the 4th ACM Web Science Conference (WebSci’12). Personality and patterns of Facebook usage. In Proceedings of the 20th Configuration Workshop, Graz, Austria, September 27-28, 2018. Liquid democracy in group-based configuration. In Proceedings of the 26th Conference on User Modeling, Adaptation and Personalization (UMAP’18). Springer International Publishing, Oxford, UK, 368–377. Beyond item recommendation: Using recommendations to stimulate knowledge sharing in group decisions. In Revised Papers from the International Workshops OHS-7, SC-3, and AH-3 on Hypermedia: Openness, Structural Awareness, and Adaptivity.
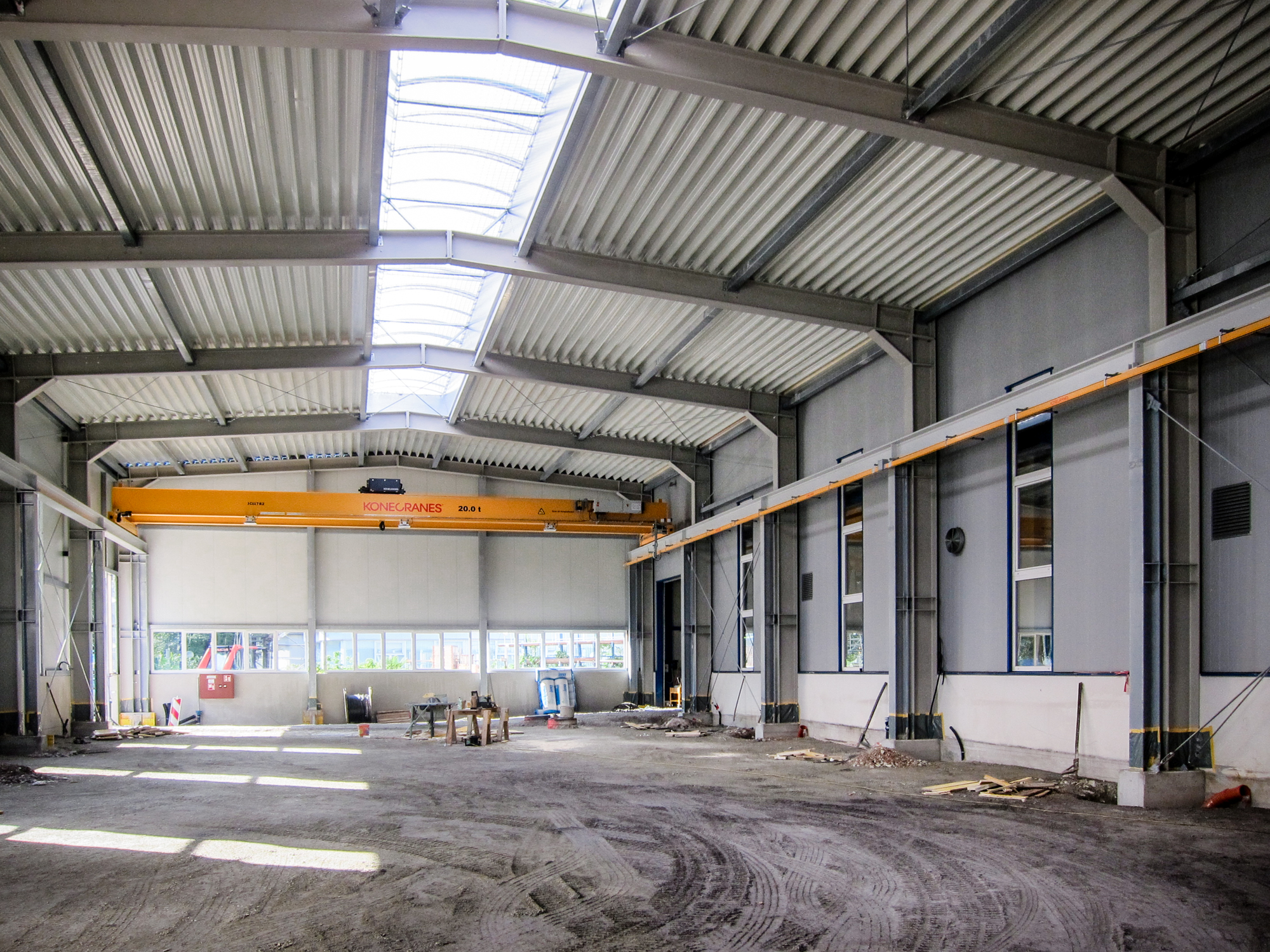
Tailoring the recommendation of tourist information to heterogeneous user groups. Group recommendation: Semantics and efficiency. Toward the next generation of recommender systems: A survey of the state-of-the-art and possible extensions. Do recommender systems manipulate consumer preferences? A study of anchoring effects. De-biasing user preference ratings in recommender systems. An emotion-aware personalized music recommendation system using a convolutional neural networks approach. In Proceedings of the 11th ACM Conference on Recommender Systems (RecSys’17). Controlling popularity bias in learning-to-rank recommendation. The provided examples go beyond single-user recommendation scenarios by also considering specific aspects of group recommendation settings.
#H graz servicecenter how to#
We include working examples to provide a deeper understanding of how to take into account these factors in recommendation processes. These factors are not only considered in single-user recommendation scenarios but, importantly, also in group recommendation ones, where groups of users are involved in a decision-making process. In this article, we provide a rigorous review of existing research on the influence of the mentioned psychological factors on recommender systems. These biases can result in low-quality decisions. Decision biases often occur in a recommendation process, since users usually apply heuristics when making a decision. In scenarios where decisions are made by groups (e.g., selecting a tourism destination to visit with friends), group composition and social connections among group members can affect the outcome of a group decision. Hence, integrating these factors into recommender systems can help to better predict users’ item preferences and increase the satisfaction with recommended items. Personality and emotions of users have strong connections with their interests and decision-making behavior. These factors are also prevalent in the existing literature related to the inclusion of psychological aspects in recommender system development. Psychological factors such as personality, emotions, social connections, and decision biases can significantly affect the outcome of a decision process.
